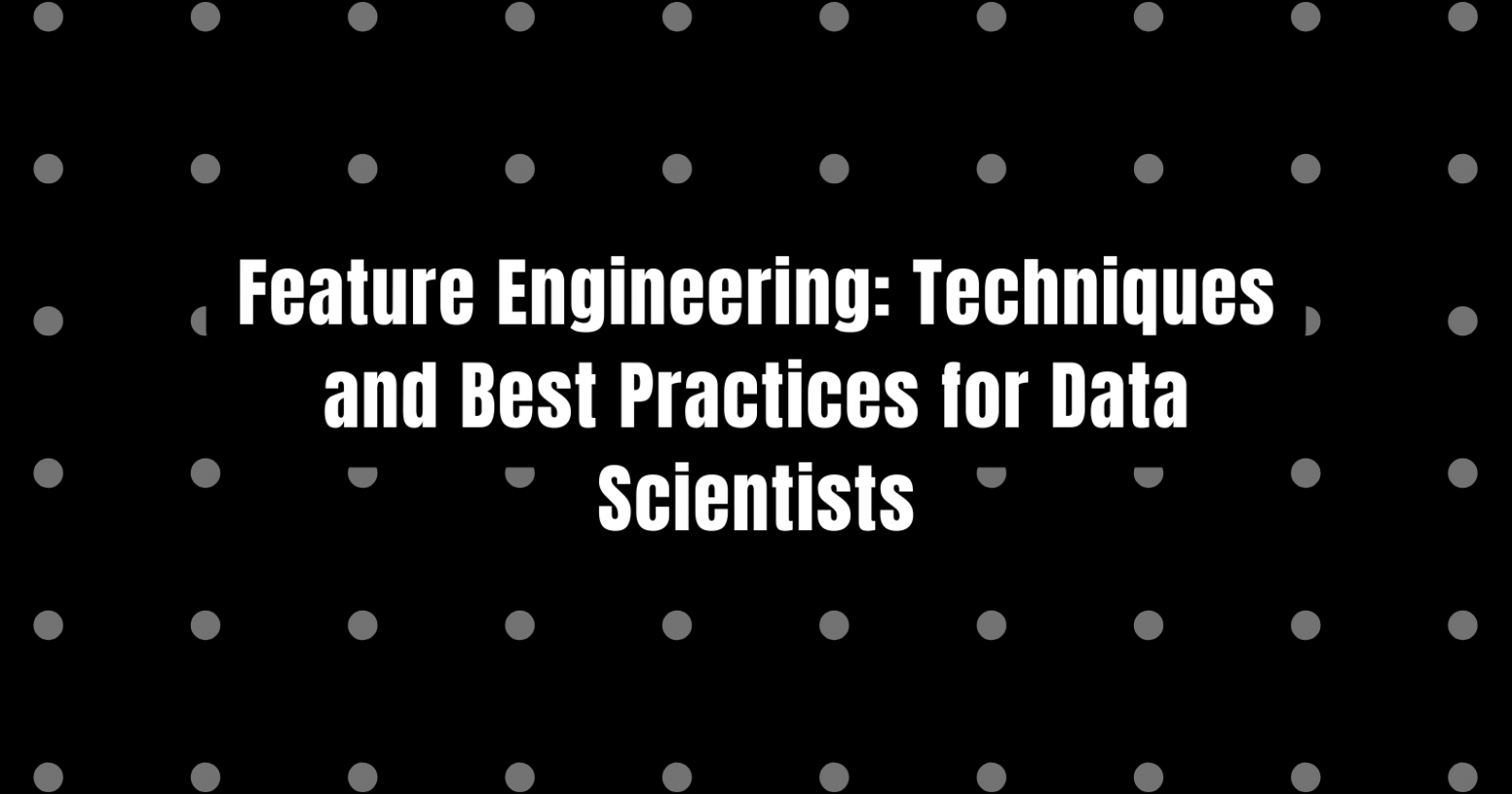
The most important stage in the data science process is feature engineering, which entails turning raw data into useful features that might enhance the performance of machine learning models. It calls for creativity, data-driven thinking, and domain expertise. Data scientists can improve the prediction capability of their models and find hidden patterns in the data by choosing, combining, and inventing relevant features. Handling missing data, scaling features, encoding categorical variables, constructing interaction terms, and other procedures are examples of feature engineering techniques. The best practises involve investigating the data, testing and improving features iteratively, and applying domain knowledge to draw out important information. The accuracy and effectiveness of machine learning models are significantly influenced by effective feature engineering.